Brain Tumor Detection Using MRI Segmentation
Brain Tumor Detection Using MRI Segmentation
- Technology : Artificial Intelligence, Deep Learning, Image Processing & Computer Vision
- Industry : Healthcare

Business Problem
- Medical imaging plays a pivotal role in diagnosing and planning treatment for brain tumors, which affect millions of individuals globally
- Accurate segmentation and classification of brain tumors from medical images are crucial to assist clinicians in understanding tumor characteristics
- The objective of this case study is to explore the application of 2D Image Segmentation using the UNET architecture for brain tumor classification and segmentation
- Accurate tumor segmentation and classification are critical in the medical field as they allow clinicians to delineate tumor boundaries, quantify tumor burden, and identify tumor subtypes
- Automated segmentation and classification methods can significantly reduce the time required for manual analysis
Solution
- To achieve this objective, we leverage the Medical Decathlon dataset, a widely used benchmark dataset designed for evaluating state-of-the-art methods in medical image analysis
- The dataset covers various brain tumor types (e.g., gliomas, meningiomas, pituitary tumors) and includes multi-modal MRI images with expert pixel-level annotations for tumor regions
- With patient metadata and meticulous annotation review, the dataset allows for consistent evaluation, insights into patient-tumor relationships, and optimal model performance through common preprocessing steps
- The UNET architecture, with its unique U-shaped encoder-decoder structure, has proven highly effective in medical image segmentation tasks
- The UNET architecture follows an asymmetric U-shape design, where the encoder path captures the high-level features of the input image. In contrast, the decoder path uses this information to generate a pixel-wise segmentation map
- The skip connections between corresponding layers of the encoder and decoder help preserve spatial information and assist in accurate localization
Result
- By leveraging the Medical Decathlon dataset and employing evaluation metrics, we have quantitatively assessed the performance of the model and visually analyzed the segmentation outputs
- The quantitative results, including the Dice coefficient, IoU, and other evaluation metrics, have indicated a high level of overlap between the predicted tumor regions and the ground truth annotations
- This demonstrates the model's accuracy and reliability in segmenting brain tumor regions from MRI scans
- The UNET architecture's encoder-decoder design, along with skip connections, has proven effective in capturing both local and global context information, leading to precise and detailed segmentation results
- The system holds significant potential in assisting clinicians with accurate tumor delineation, enabling better diagnosis, treatment planning, and monitoring of brain tumors
- Our case study has showcased the efficacy of 2D Image Segmentation using the UNET model for brain tumor classification and segmentation
- The model's ability to accurately identify tumor boundaries and classify tumor regions holds great promise in improving clinical decision-making and patient outcomes
Potential Paths For Future Research And Development
- 1. Integration of multi-modal data: Investigating additional imaging modalities, such as diffusion-weighted imaging (DWI) or perfusion imaging, can provide complementary information and enhance the model's performance in characterizing brain tumors.
- 2. Incorporating clinical metadata: Integrating patient demographics, genetic information, or other clinical data into the segmentation model can assist in personalized tumor analysis and treatment planning.
- 3. Transfer learning and fine-tuning: Using pre-trained models on larger datasets, such as ImageNet, and adapting them to the brain tumor segmentation task through fine-tuning can enhance the model's performance.
- 4. Robustness to variations: Investigating the model's performance across different acquisition settings, scanner types, and imaging protocols can provide insights into its robustness and generalizability.
- 5. Real-time inference: Optimizing the model for real-time inference on resource-constrained environments, such as mobile or embedded devices, can facilitate its deployment in clinical practice.
The insights gained from this study have the potential to contribute to the advancement of medical imaging analysis and aid clinicians in making informed decisions for brain tumor diagnosis and treatment.
Note: While our case study focused on the Medical Decathlon dataset, the findings and methodology can be extended to other datasets and clinical scenarios. Further research and validation on diverse datasets are necessary to assess the model’s generalizability and robustness in real-world clinical settings.
Connect With Us To Leverage Data For Insights And Intelligence
Case Studies
Crafting Data Success Stories
As your reliable data partner, we empower global businesses to seize opportunities and drive impactful change
Secure Data Migration Journey For A Leading Construction Company
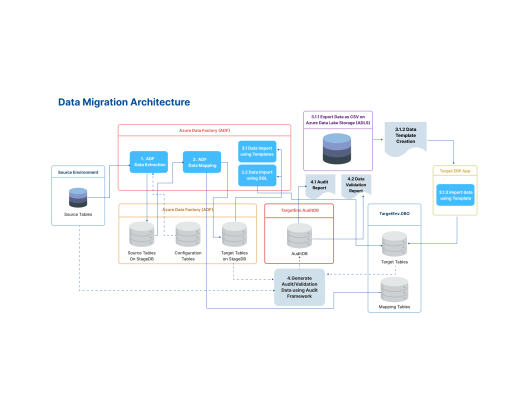
QlikView To Microsoft Power BI Migration
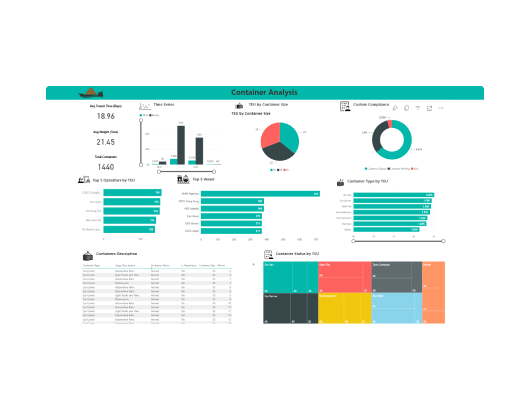
Comprehensive Healthcare Survey Application with Omni-Channel Capabilities
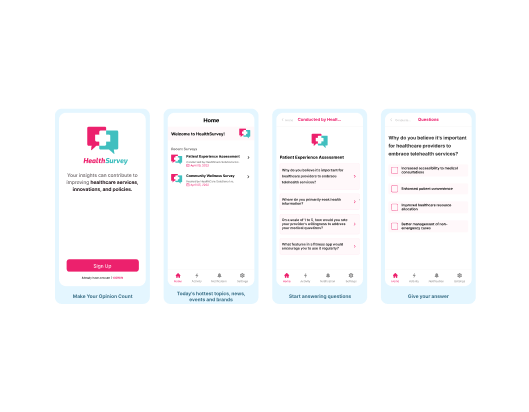
Testimonial
Our Source of Motivation
Excellent to work with in every way. Proactively identified solutions to the problem in the initial design and the recommended solutions. Work has top-notch. Results delivered on time. Communication was excellent.
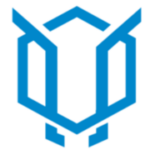
Real pleasure consulting with Ridgeant to set up our company's entire data warehouse and dashboards on AWS. I will definitely be reaching out to them for future work to be done. Our project was effective and 100% achieved what I planned to do in the beginning, in a shorter time frame and with less effort than I expected.
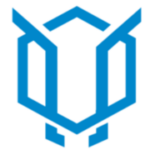
CCR Data perform complex data migrations, we needed and extra pair of hands to restore an Oracle database and transfer the data to a Microsoft SQL database ready for our migration analysts to do their stuff. Nitesh was able to make available at short notice one of their team who was able to see the project through from start to finish. We would not hesitate in recommending or using Ridgeant again and would be happy to outsource bigger projects to them in the future.
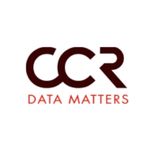
I have used RA on numerous occasions over the past 2 years, specifically with Nitesh Solanki for the delivery on PDI ETL jobs. I am very happy with him and the high level of quality work he has provided. He seems to be available all the time and works extremely hard to deliver high quality solutions.
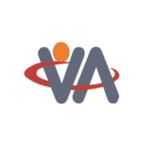
Working with Ridgeant was a game-changer for us. Thanks to Nitesh from Ridgeant, our data strategy is on point and giving our business a major boost!
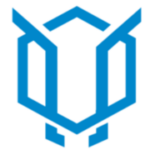
They took us from square one, building a smart data strategy – everything from collecting data to dishing out real-time insights. With their help, we've seen some major improvements. We would give them a thumbs-up for anything data-related.
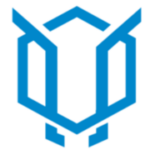
Ridgeant has made a real difference for our insurance firm. Their know-how in fraud detection is top-notch. Their data strategies have been a big help, and we are seeing great results. We are quite pleased with what they've done for us
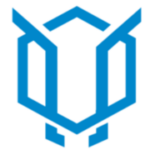
They truly understand what they do. Their restaurant analytics provide real-time insights into our operations and customer behaviors, and it has made a significant difference in our business.
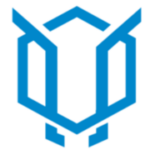
Working with Ridgeant has been fantastic! Thanks to their AI and ML-powered solution, we've made great progress. Their expertise helped us spot and prevent fraud in rentals and make us trustworthy.
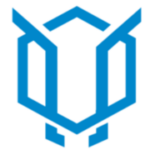
Previous
Next