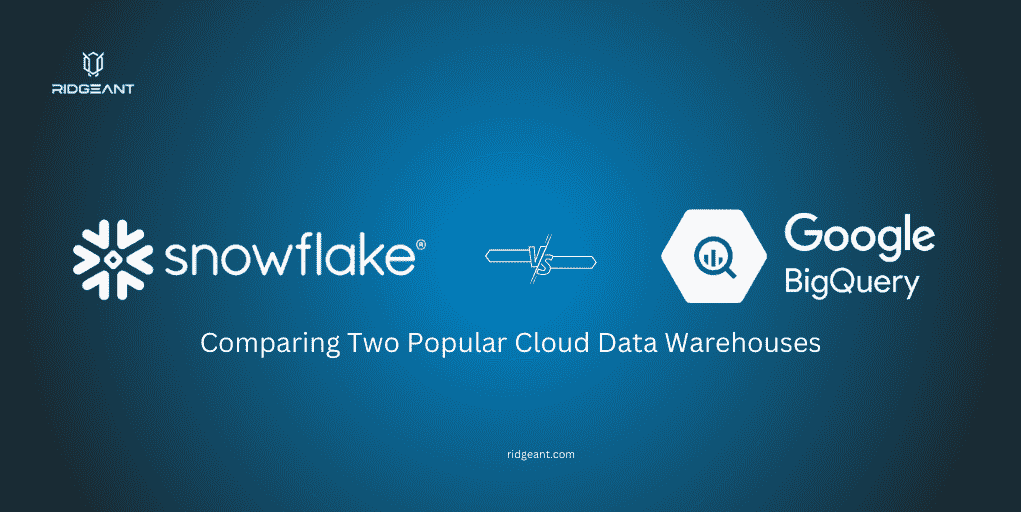
Snowflake vs BigQuery – Comparing Two Popular Cloud Data Warehouses
- Snowflake, Data Science, Cloud
- August 28, 2023
- Ridgeant
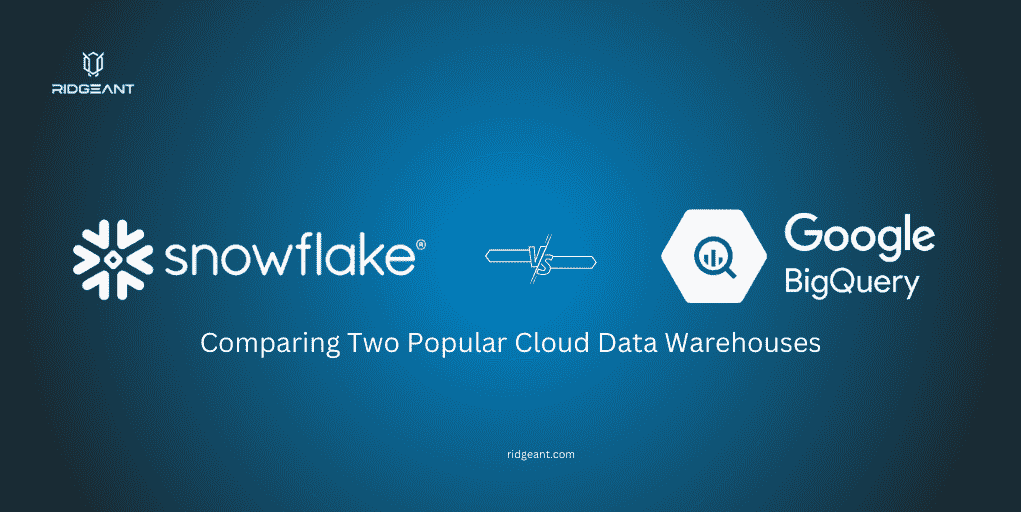
As organizations lean on the massive volume of data that is being accumulated, for insightful decision-making, the apt choice of a cloud data warehouse is important. Project owners take time to decide on which one to select since it directly impacts the power to transform business through data-driven insights.Cloud-driven data storage and processing is the key to business success now and hence business owners and data architects must choose the best fit for a successful output. When it comes to cloud-based data warehouses, two popular names that suit the bill are Snowflake and Google BigQuery.Often compared with each other, Snowflake vs. BigQuery offers an interesting set of parameters against which they can be evaluated. Through this article, we offer a detailed assessment of the two tech stalwarts, to help you finalize which one is best for your organization. Before comparison, let us delve into the overview and features of each.What is Snowflake?Snowflake is a popular platform that powers the data cloud. You can execute your most critical workloads on top of Snowflake’s multi-cluster shared data architecture in a fully managed platform that capitalizes on the near-infinite resources of the cloud.Snowflake, as a SaaS platform, offers a three-layered architecture having the best of shared-nothing and shared-disk models. The three layers are the data storage layer, the query processing compute layer, and the cloud services layer. Data warehouses in Snowflake can be hosted on AWS or Azure.It makes use of an SQL database engine with a cloud-driven architecture and hence is easy to use and fast to operate. The compute and storage needs are separated and hence there is scalability and flexibility of resources. Fit for modernized data workloads, it is a fully managed technology and has a serverless architecture.
Key Features of Snowflake
- Fast and elastic scalability
- Security and compliance with standards
- Cost-effective with a pay-per-use model
- Supports other cloud service providers
- Well-designed data manipulation services
- Robust query profiler
- Delivered as a Service
- Complete ANSI SQL database
- Unlimited query concurrency
What is BigQuery?
BigQuery is a serverless and cost-effective enterprise data warehouse that works across clouds and scales with your data. Powered by Google, BigQuery is a robust cloud data warehouse that is serverless and fully managed. It makes use of a columnar storage format for analytical workloads. It integrates seamlessly with other GCP products.Dremel is an effective query engine that is leveraged for query execution in BigQuery. This interactive query system segregates the complicated queries into nested, smaller components which can then be processed individually and reset for a unified result. BigQuery also uses Colossus for data replication and recovery, Jupiter for distributed computing and storage, and Borg for cluster management.It supports different data formats that are structured, semi-structured, or unstructured like CSV, AVRO, Parquet, JSON, etc. It executes in a multi-tenancy mode with shared resources that are given as slots representing virtual CPU executing SQL.Key Features of BigQuery
- Inbuilt Machine Learning integration
- Low latency streaming
- No requirement for the provisioning of servers
- Scalability to manage large datasets
- Easy integration with other GCP products
- Geospatial analysis
- Access to unsampled raw events and user-level data
- No cardinality limits
- Multi-cloud functionality
Comparing Snowflake vs. BigQuery – The Similarities and Differences
The Similarities- Secure, scalable, and powerful data warehouses
- Columnar storage and Massively Parallel Processing (MPP)
- Cost-driven query planning
- Decoupled storage and compute resources
- Low maintenance burden with higher usability
- Supports key-pair authentication, multi-factor authentication (MFA), single sign-on (SSO), and OAuth 2
- Compliant with industry-specific regulations like HIPAA
- Support for materialized views
- End-to-end data encryption
- Supports customer-managed encryption keys (CMEK) for better control over data encryption
- Compatible with varied third-party tools for transforming, visualizing, and analyzing data
- Easy ingestion and replication of data
Quick Comparison: Snowflake vs. BigQuery
Snowflake | Big Query | |
Technical Proficiency | Fit for teams with multiple data tools and cloud services experience | Fit for teams well known to Google Cloud Platform and other GCP services |
Scalability | 8 concurrent queries for each warehouse, autoscaling till 10 warehouses, independent scaling of compute resources | Restricted to 100 concurrent users by default, serverless architecture, automated resource allocation |
Architecture | Multi-cluster shared data architecture, decoupled compute and storage architecture permitting ad-hoc resources. | The serverless architecture permits ad-hoc resources without having to bother about computing, simplifying resource management based on query requirements |
Performance | High performance because of its architecture and automated query optimization | High performance because of its faster queries, columnar storage, caching styles, and Dremel |
User Management and Access Control | Robust access control through role-based access with support for Single Sign On (SSO) | Robust access control through GCP’s identity and access management (IAM) system |
Concurrency and Workload Management | Multi-cluster computing and scaling fit in concurrent queries and users | Serverless design allocates resources in an automated way, with more concurrency |
Supported Cloud Technologies | Azure, AWS, Google Cloud | Only Google Cloud |
Table Level Partitioning | Automatic division of data in micro partitions with cluster keys and pruning | Users with pruning define partitions at the table level at the partition level |
Network Security | Restricts virtual private networking to those who have a subscription to a Virtual Private Snowflake edition | Permits all Google Cloud Platform users to use a virtual private network through GCP Virtual Private Cloud Service Controls |
Pricing Model | Makes use of a time-based pricing model for computing resources | Makes use of a query-based pricing model for computing resources |
Query Performance | High-performance query execution and automated query optimization | Faster query performance through columnar storage and caching methodologies |
Warm Cache (SSD) | Yes | No |
Data Connectors | Native data connectors with third-party integrations | Smooth integration with other GCP services |